
Loading ......
Technology laboratory for Enterprise Artificial Intelligence

Inferz: Rethinking AI
Our ground-breaking AI software platform enables the building of next generation AI applications which can self-learn and self-reason with an inherent intelligence to handle the multiple conflicting uncertainties and complexities of the real world. The applications are based on dynamic discovery of knowledge and common-sense reasoning, are fully transparent and are able to communicate conclusions reached by analytic and synthetic acumen.
The platform has been built bottom up on the principles of soft computing, perpetual reasoning and model driven cognition.
Our cognitive platform enables a new generation of AI applications delivering understanding and advanced problem-solving in ambiguous real-world settings
Cognition not just Recognition
Mission
Inferz is an AI Technology Laboratory. We are focussed on innovation in cognitive AI.
Our mission is to develop a new generation of symbolic/sub-symbolic AI which overcomes the limitations of existing AI. This new generation is characterised by AI which can both self-learn and self-reason (not just one or the other), is transparent (not black-box), can tolerate the multiple forms of complexity and uncertainty inherent in the real world (not failing when confronted by the unexpected, the imprecise or confliction) and can deeply understand natural language (not just statistically match or detect pattern features).
We develop new forms of representations, algorithms and technologies - unifying symbolic and sub-symbolic approaches to solving problems or synthesising solutions in pervasively uncertain situations.
Causation not just Correlation
The Inferz platform unifies machine learning, machine reasoning, machine-based language understanding and other capabilities.
It is based on the principles of soft computing (i.e. is tolerant of imprecision, uncertainty, partial truth, multiple contexts, approximation and likelihoods), perpetual reasoning (i.e. continuous and recurring revisions of belief) and model driven cognition (i.e. using comprehensive ontologies and knowledge to reason and learn), and employs novel forms of representations, algorithms and technologies. As a whole, the Inferz platform unifies symbolic and sub-symbolic approaches to solving problems or synthesising solutions under pervasively uncertain situations.
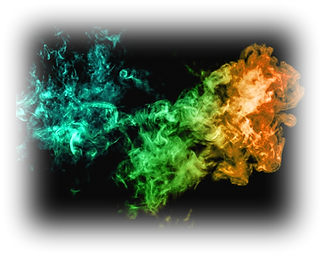
Inferz’s principals each have four decades practical experience of applying a wide range of AI techniques to deliver a broad spectrum of business benefits.
We are supported by a network of associates drawn from academia and business who are thought leaders and innovators in the application of Adaptive AI to complex problems.
We continue to build our network of participators and advisers who wish to be part of a community to further the cause of true cognitive AI. Please contact us if you wish to take part.
Technology
Team
Soft Cognitive
Computing
Scroll down
for more
Motivation
Inferz is our response to many years’ first-hand experience of implementing AI. For each wave of AI we have encountered major limitations. In particular, we believe that the current wave of Neural Network based AI has hit a brick wall because it is no more than opaque pattern matching without any ability to reason or understand. Our AI platform draws on elements of earlier waves, harnesses modern computing power (which was not available to the early waves of AI) and adds new elements (see below).
Our motivation is to build better AI. We operate as a Technology Laboratory with no plans to productise our platform.
At some point we will work with other parties to ensure that the potential of the Inferz platform is fully realised.
A mandate for an adaptive cognitive AI
Current AI is in a logjam.
The simplicity of ‘connectionist AI’ was introduced to overcome the lack of computing power required for the ‘smart AI’ of the 80s/90s. But connectionism has led AI into a simplicity tar pit because of its lack of transparency, symbolic representation and acumen. Ironically computing power has now surpassed the dreams of earlier AI practitioners and symbolic AI.
In the 90s the AI community faced a dilemma. We needed to increase the sophistication of AI capabilities to address increasingly ambitious real-world analysis/synthesis tasks, whilst being progressively thwarted by the lack of computing power. Unfortunately, and with hindsight, the AI community made a poor choice - we opted for overly simplifying the world and building an AI momentum on a cartoon of AI, one based on simple recognition technologies. This is motivated by a grossly simplified interpretation of the human brain’s neural structure (connectionism). This constrained problem solving to just detection processing and simplistic pattern learning. To handle real-world complexity AI requires much more including cognitive processing and sophisticated adaptive/dynamic reasoning. The failure to appreciate that the ‘emperor has no clothes’ is why we are now in a logjam. Non-symbolic neural recognition AI will never be able to realise cognitive computing ambitions. Replicating the mind (not just the brain) requires the power of symbolic cognition in combination with the current generation of power computing.
Such higher-level acumen needs to deal with the ‘polyform’ nature of our complex world. An AI is needed that handles the complexity of human-like learning (ML) and reasoning (MR), explicit representation, uncertainty/vagueness/probability, breadth of dynamic truth/belief. It should be capable of handling: the hyper-dimensional nature of learning/reasoning in complex worlds; the hype-associations, relations, sequences and the extra-dimensional facets of time and events; and the meta-dimensionality properties of world such as context, conjecture, hypotheses, assumption, etc. Polyform AI also recognises and handles the myriad forms and types of insight, wisdom, knowledge and information.
But we are now overstretching ‘naïve AI’ beyond its capabilities. It is a recognition technology ill-suited to cognitive machine thinking. What is required is a new mandate to re-engage smart symbolic AI and move forward towards cognitive intelligence whilst using some of the restricted advances of non-Symbolic/sub-Symbolic AI.
Highlights from the progressive AI mandate are shown below, the full mandate can be viewed by partners using the link.
Fundamentals of adaptive cognitive AI
Soft computing is the cognitive basis for analytics and synthetics
Soft computing is fundamental to cognitive AI as it is able to deal with vague and probable forms of knowledge, situations, reasoning and learning, and to manage simultaneous multiple contexts/motives in an adaptive way. Soft computing differs from conventional (hard) computing in that soft computing is tolerant of imprecision, uncertainty, partial truth, approximation and likelihood.
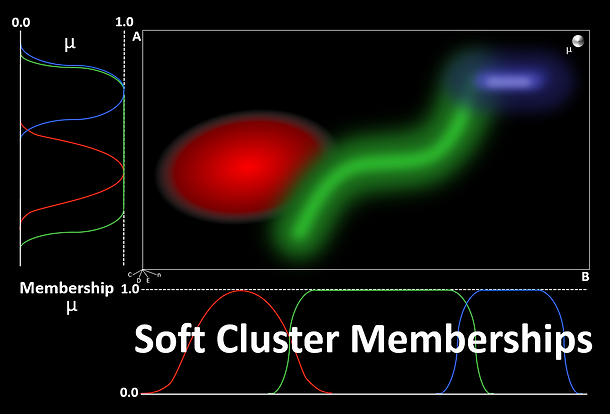
The guiding principle of soft computing is: exploit the tolerance for fuzziness, vagueness, likeliness and holding multiple contradictory beliefs, ideas, or values at the same time. Soft computing is exploited by learning, reasoning, representation, retrospection and other AI capabilities. Being able to deal with the vagaries of the real world delivers tractability, high resolution, high precision and robustness in solution modelling and problem solving as required in many areas of complex enterprise systems.
A summary mandate for an adaptive cognitive AI
The following introduces each section of our mandate (with a more extensive explanation of each section available using the 'more' button). Some of the details require a user login to view the material. The full mandate is available to partners.
An important mandate concept is unified soft computing. Cognition is founded on graceful soft computation which is a merging of soft machine learning, soft reasoning, soft optimisation, soft knowledge representation and soft linguistics. These elements are interplaying throughout the soft framework technology.
Uncertainty tolerance through soft computing
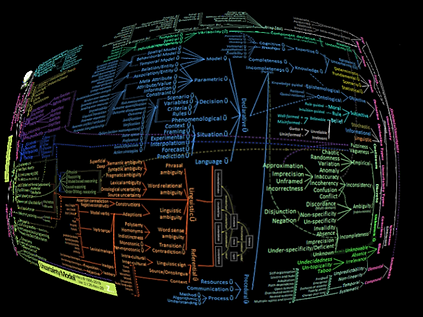
At its core AI is a process of reducing uncertainty in situations. AI algorithms and representations must be able to intelligently handle complex real world-situations. They must also be able to reason with significant imprecision, vagueness, multiple truths and a ubiquitous breadth of varied uncertainties to be able to solve problems or predict consequent world states. Such an AI is required in cognitively complex domains (e.g. human behaviour, forecasting, logistics, prediction, etc). Recognition based AI is not sufficient; what is needed is AI with adaptive comprehension and understanding of all the realms of uncertainty.
In contrast to many of today’s AI technologies, which sweep polyform uncertainty ‘under the carpet’, we promote the use all the varieties and sources of uncertainty in the cognitive process to emulate the subtlety of acumen exhibited by human intelligence. Our platform has a foundation of analogy reasoning and learning which exploits advanced rationalising technologies to enable graceful cognition.
Soft computing is fundamental to cognitive AI as it is able to deal with vague forms of knowledge, situations, reasoning and learning, and manage simultaneous multiple contexts/motives in an adaptive way.
Mandate (Generic NoScroll)
Public
To view more details:
please view on a desktop
RealmsOfUncertainty
Cognition not just Recognition
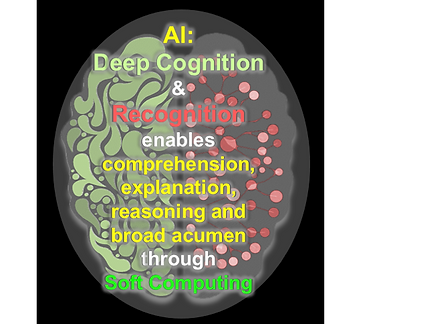
Cognition is acquiring knowledge, understanding and truth through thought, experience, the senses and also partially through recognition. It is the combination of knowledge and reasoning to predict new implicit unobserved insights (data, facts, information, knowledge, wisdom, etc). This involves comprehending and understanding the world using inherently symbolic representations and processes.
In contrast, recognition is the acknowledgment of something's existence, validity, or legality. It is essentially labelling an ‘item’ with a ‘symbol’ through superficial pattern recognition. This can be either a sub-symbolic or a non-symbolic process, it does not need the impenetrable contortions inherent within neural computing.
Explanation, justification and transparency of insights are by their very nature fundamental methods for cognitive and language-based processes.
In contrast to many of today’s machine learning algorithms which are focused on just recognition, Inferz is focused on human-like cognition capable of reasoning under uncertainty with multiple world views. Inferz’s technology is capable of both soft pattern-based recognition/perception and more powerful language/knowledge-based inference i.e. representational cognition.
Mandate (Generic NoScroll)
Public
To view more details:
please view on a desktop
TrueCognitiveAI
Explainability and Justification
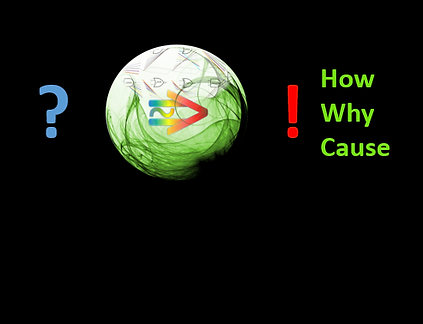
Inferz’s algorithms/representations are fully justifiable (it is clear exactly how and why each conclusion is reached). Both procedural and causal explanations are available. This is of particular importance in fields such as medicine, engineering and law, where auditability is required for practical or regulatory reasons.
Each cognitive decision or situation state clarification is justified by:
-
facts and certainties that are associated with the current contexts
-
real-time working model information
-
domain models (ontology based)
-
machine learned experience held in the knowledge bases
All of these sources are used to draw conclusions, explanations, justifications, levels of certainty, causalities and clarifications via machine reason-based auditing inference.
Connectionist AI has only reached the simplest stages of recognition, equivalent to the neural power and awareness of a slug. It will always be limited to recognition tasks only. It is not self-aware, because its processing is simplistic, obscure and opaque with no representational decision method, which is essential for justification. As such it is impossible for connectionist AI to explain or clarify itself. Even more crucially it has no human-related model of the world to articulate ‘why’ or ‘causation’ to a human. It has no meta-knowledge, understanding or meta-cognition.
Mandate (Generic NoScroll)
Private
To view more details:
please view on a desktop
ExplainAndJustifyAndTransparent
Sophisticated soft polyform machine learning
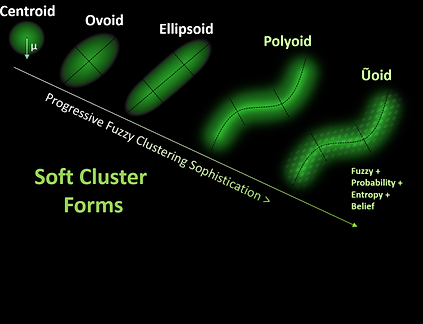
Polyform learning is a holistic approach to machine learning which is able to utilise all forms of uncertainty and complexity.
Fundamental to its success is the soft computing principle of combining several treatments of uncertainty as an intrinsic part of learning and synthesis.
There is a need to incorporate the following approaches to handle the complexity of human-like learning: fuzziness/approximation, probability/likelihood, belief/truth, entropy/information-disorder, etc. Machine learning engines typically have only one method of handling uncertainty or none at all: in either case this results in naïve AI.
Along with the handling of multiple forms of uncertainty, machine learning engines must also be able to: test the validity of the resultant synthesised knowledge through understanding ontological sense, explain the source of information from data, explain the derivation of that knowledge and explain the derivation of predictions from that knowledge.
Mandate (Generic NoScroll)
Private
To view more details:
please view on a desktop
SoftMachineLearning
Multiplicity of reasoning for powerful understanding
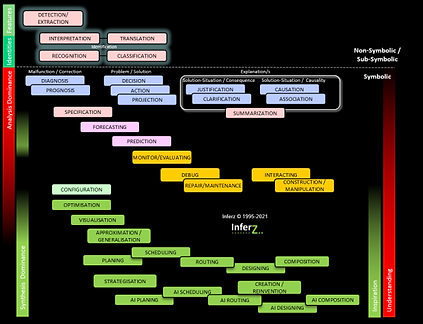
Machine learning can be a powerful knowledge synthesis technology, but without machine reasoning the AI system will only execute/invoke previously synthesised patterns. They will only perform recognition not true cognition. They will lack the ability to infer or create unseen implicit situation consequences.
Such reasoning should be able to fully discern the consequences of subtle uncertainty (i.e. fuzziness, probability, bias, belief, truth, etc) in the world. AI limited to only machine learning will merely achieve ‘idiot savant’ status in limited domains and simple consultation paradigms. Such AI will only appear intelligent, like a Victorian automaton – fascinating but fake.
Instead AI needs to be able to solve deep complex problems/situations, it needs to be dynamically adaptive using a variety of reasoning techniques (i.e. inference: deductive, inductive, abductive, etc; also theorem provers, constraint satisfiers, linguistic reasoners, etc) and to be aware how/when to invoke each technique. It needs to recognise entities/situations but it also needs to understand, be aware and be astute to attain true cognitive capabilities.
All this requires explicit symbolic reasoning - logical, non-logical and knowledgeable. The reasoning process should be fast and scalable, exploiting modern computation power, parallel processing and coherence-based computing.
Mandate (Generic NoScroll)
Public
To view more details:
please view on a desktop
HighLevelConsultationalParadigms
Combined soft and polyform AI

Polyform cognitive processing is a holistic approach to both machine learning and machine reasoning (ML/MR). Fundamental to cognitive ML/MR’s success are the principles encompassed in soft computing, i.e. utilising multiple treatments of uncertainty as an intrinsic part of representing, learning, discovering and subsequent predicting. Polyform AI combines and incorporates the following technologies to handle the complexity of human-like ML/MR: fuzziness/approximation, probability/likelihood, belief/truth, randomness/entropy and others.
Polyform AI recognises the hyper-dimensional nature of ML/MR, not just the multiple attributional nature of the problem space and its curvature, but also the associations in the space - degree of set membership, the probability of set assignment, the degree of belief in the set information; the hyper-associations/relations/sequences present in the space; the extra-dimensionality facets of time, events and the like; and the meta-dimensionality properties of the solution search space such as context, conjecture, hypotheses, assumption, etc.
Polyform AI also recognises a myriad of forms and types of insight, wisdom, knowledge and information.
Mandate (Generic NoScroll)
Public
To view more details:
please view on a desktop
CombinedSoftAndPolyformAIDetail
Transparency and contextual truth: multi-layered

In addition to explainability and justification, a real-world AI requires:
-
Transparency – not only making explicit the derivation of the facts in the world model/situation but also defining the different forms and magnitudes of uncertainties in those facts, decisions and observations. Such uncertainties include – bias, approximation, likelihood, and others.
-
Context of truth, a maintenance of truth and the degree of assumptions in the truth.
Transparency should involve all the artefacts of reasoning (and learning) from explicit content, data and information, derived implicit data/information/facts, and the invoked knowledge/wisdom/insights and how they are utilised.
These artefacts are multifarious and have context and uncertainties accompanying their use in the reasoning and broader cognitive process.
Mandate (Generic NoScroll)
Public
To view more details:
please view on a desktop
TransparencyAndContextualTruthDetail
Graceful cognition: approximate and qualitative reasoning
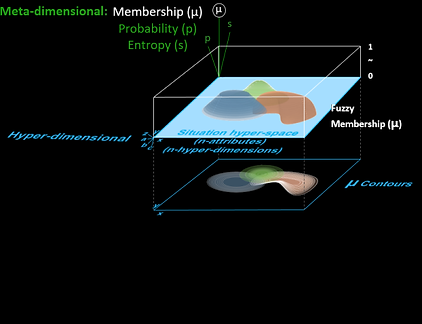
Vague rationale, as embodied in approximate (and probabilistic) reasoning, results in solutions that avoid the false beliefs and oversimplifications inevitable in Boolean/Bayesian hard logic systems.
Progressive qualification and endorsement of decisions under uncertain or ambiguous facts/knowledge is how humans deal with the dynamic shifting nature of reality. Also the real world is not precise or cut-and-dry: it is a complex world that naïve neural recognition technologies oversimplify, leading to brittle problem solving.
Cognition is by its nature able to operate with vague inference when dealing with intricate, imperfect and imprecise continuums of understanding that are all-pervasive in the world and how it is represented.
AI must be able to reason and learn with progressive gracefulness as the world gets more imprecise, indiscrete and indeterminate.
Mandate (Generic NoScroll)
Private
To view more details:
please view on a desktop
GracefulCognitionDetail
Soft ontology driven discovery and prediction

The world and its problems/opportunities are easier to understand if they can be conceived on the basis of an underlying model. Such a model should be representational and have sufficient erudition for the performance of cognitive tasks and be complementary to language-based elucidation. This model should be explicit but conceptualised from first principles that can encompass both competing ideas and many aspects of uncertainty – whether they are intuitive or counterintuitive. This model should be a repository of ontological forms of knowledge. Based on an intrinsic core ontology, it is required that machine classification of general/domain centric content should continuously refine/discover new ‘extrinsic’ ontological items, associations and uncertainty characterisations.
Soft machine learning is an ideal method for generating the ontology using fuzzy clustering with probabilistic and entropy-based metrics. These metrics should guide the characterisation of the linguistic entities and soft semantic networks. Polyform fuzzy clustering and comparative soft truth maintenance is ideal to enable the autodidactic learning and discovery of ontological knowledge.
The ontology will be the underlying world model for focusing general/domain/language-based reasoning using conditional knowledge that has been garnered by sub-symbolic and symbolic soft machine learning.
Mandate (Generic NoScroll)
Public
To view more details:
please view on a desktop
SoftOntologyDiscoveryAndPredictionDetail
Linguistic and soft language-based cognition

Cognitive AI requires a move from the simplistic in-vogue statistical based Natural Language Processing (NLP) to embrace Natural Language Understanding (NLU). Using statistical NLP we lose all context and meaning resident in the textural content as it is converted into just data about the words. We lose all semantic meaning and the nuance inherent in the imprecision/vagueness of words or their settings. NLU based on statistical correlation loses the ability to reason deeply about language usage and world insights expressed in the ambiguity of the language.
Linguistic intelligence based around language models, world models and language-based reasoning is an adjunct to domain-based problem solving. It uses a parallel approach of understanding and reasoning about concepts framed in words. It aims at enhancing the AI’s ability to think constructively about what is being communicated and why: to think, reason and solve problems in different ways. This is very unlike simple vocabulary recognition. It leverages the intelligences held in the linguistic propositions leading to arguments and reasons by which to enhance discovery.
Language is much more than the shallow understanding of words, their meaning, their usage and their semantics. Language usage encodes not just description/enquiries but importantly the uncertainty and wisdom of a situation through the choice of words and their articulation. Crucially, the expression of vagueness/imprecision with regards to the meaning (semantics), our level of knowing (epistemic), the inherent fuzziness and likeliness due to genuine indeterminacy in the situation (ontic) and our ability to describe a situation (nomic) are a valuable insight for reasoning about the world. Currently this meta-knowledge is ignored or ill-used by non-symbolic AI. In Cognitive AI, the use of vagueness is fundamental to understanding our imperfect real world and places caveats on our levels of precision to reason about it. It is the way the human mind works. It is also fundamental to the AI’s ability to communicate such reservations in situations or solutions. Ethical AI is a direct consequence of the recognition of uncertainty and bias.
Non-symbolic statistical word matching loses all this necessary capability. What we need is an ontology-driven linguistic reasoning technology which can exploit and use the uncertainty insights bound and encoded in the written word. By adopting deep linguistic learning and reasoning, embodying the power of fuzzy logics, we can emulate common-sense and maintain complete transparency of the resultant cognitive knowledge and acumen.
Mandate (Generic NoScroll)
Public
To view more details:
please view on a desktop
LinguisticsAndSoftLanguageReasoning
Inferz mandate
In short, the Inferz mandate for AI is to replicate fully adaptive human cognitive processes: the human mind not the human brain. It is a more difficult task than the current simplistic neural technologies. Current AI has hit a road-block by merely digitally mimicking the human brain’s neural network architecture which stymies progress to General AI.
So a different approach is needed. One which is:
-
explicitly symbolic
-
strongly representational and ontological
-
underpinned by and universally driven by an understanding of uncertainty
-
can deal with both recognition and high-level cognition/problem solving
-
explicitly based on cognitive psychological tenets and process
-
can cope with the polyform nature of reasoning, learning and motivation
Such an adaptive cognitive approach is built to provide predictions in situations whilst able to deal with the inherent uncertainties in solving problems. As such it is firmly based on human vague rationale and is explicitly designed to provide sufficient explanations; it can transparently clarify a situation and why it came to be.
That is the MI.ND platform.
Cognitive AI and the Machine
Problems are not all alike: problem solving has different orders of complexity and approaches
As problems become more complex and the learning of knowledge is based on a synthesis of billions of cases, humans cannot cope. Progressively human expertise needs to be replaced by machine intelligence and machine expertise, as the world becomes more instantaneous, uncertain, complex and continuous. This machine expertise needs to become based on soft computing and derived through machine learning – manual programming is just too error prone and slow.
Human experience should be restricted to monitor and critique sampled solutions, using their conscious common sense to highlight issues in the machine behaviour.
Unfortunately, this also has limits as problem/solution complexity rises. Catastrophic failure in our overly automated world is inevitable, and this will limit progress until the next paradigm shift. Enterprises will inevitably be led into the new paradigm of problem solving with soft AI applying adaptive cognitive approaches.
A capable cognitive platform for adaptive AI applications
MI.ND: an adaptive cognitive AI platform built on standard technology frameworks and running on standard consumer cloud platforms. This proprietary platform interworks the capabilities of the AI mandated needs stated above. Inferz builds elements or all of its platform as a unified suite of cloud-services available for containerised deliverables on commercial provider platforms.
Contact our team to find out more about this new form of AI.

For a more technical architecture see our marchitecture diagram.
Inferz technologies
Inferz’s proprietary methodologies and technologies unlock a new paradigm of Unified Soft Computing and
Adaptive Artificial Intelligence for the Enterprise.
Combining the best technologies from symbolic and sub-symbolic computing. Founded on the adaptive power of soft machine learning and reasoning. Replacing the black box obscurity of neural networks with a new breed of
machine learning based on polynomial fuzzy + probabilistic discovery. It removes the ‘causal chasm’ by combining symbolic and sub-symbolic representations – excluding the opaque non-symbolic black-box by modelling the mind not the brain.
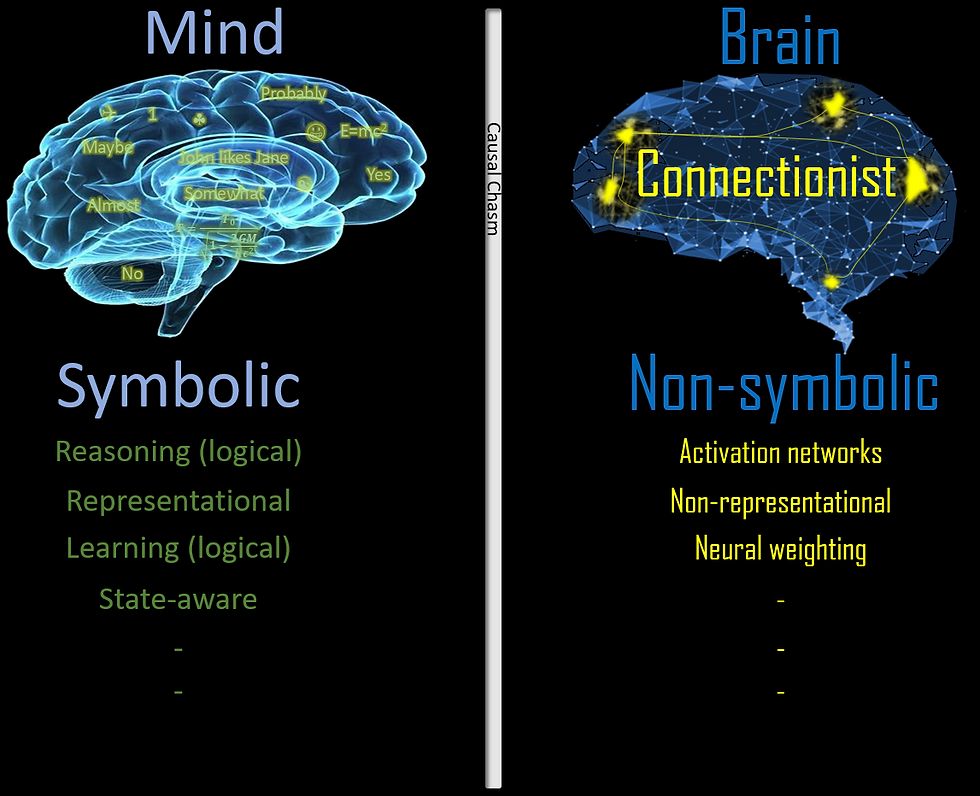
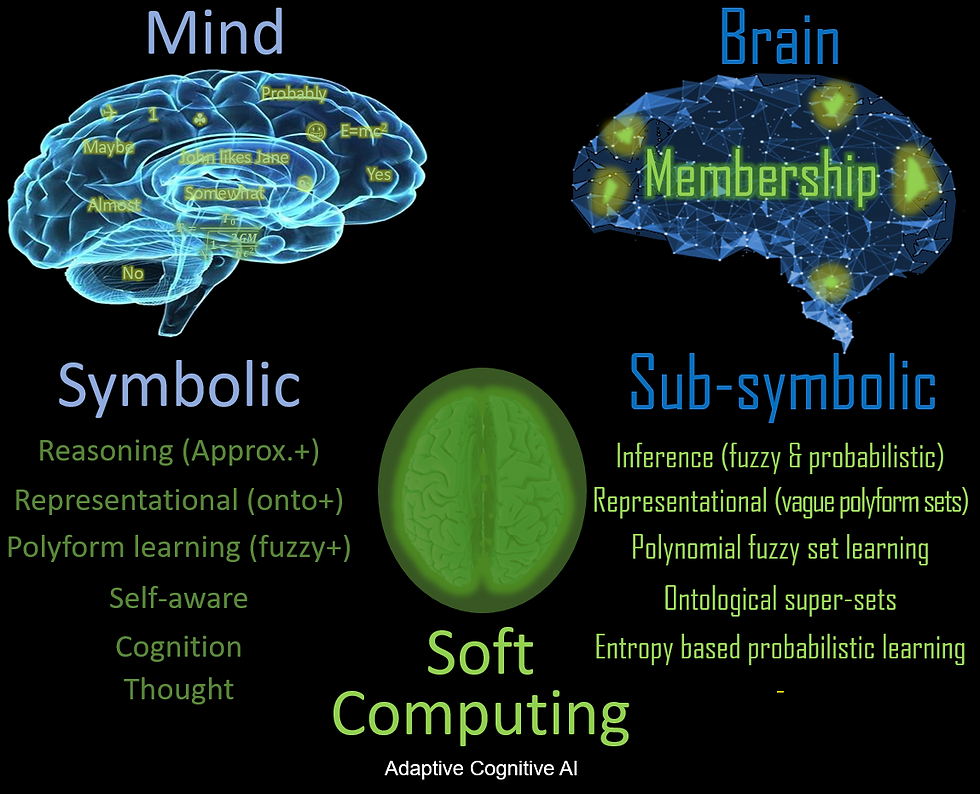
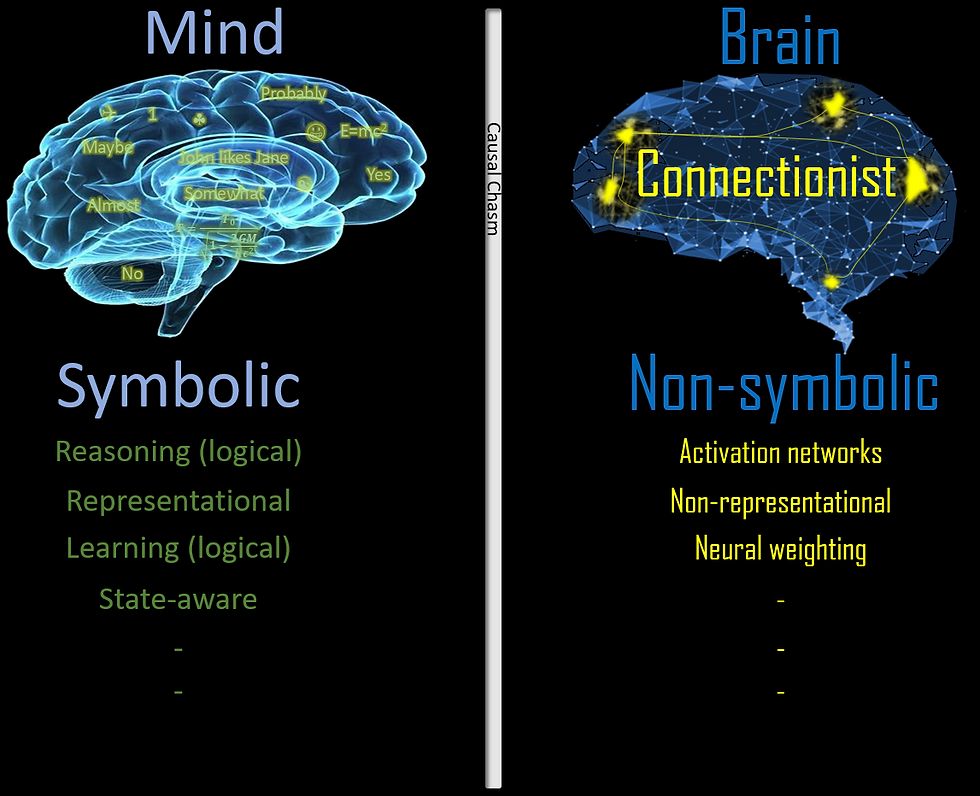
Expanding the perspective of the MI.ND architecture encompasses not just deeper cognitive technologies
but also broader hyper-cognitive technologies.
Inferz’s proprietary methodologies and technologies open the new world of Unified Soft Cognitive Computing
If you need to move to smart AI - from mere recognition to the higher levels of cognition – get in contact.
Inferz is Rethinking AI